What is lead scoring?
According to Wikipedia, lead scoring is hierarchically ranking prospects against certain objectives. The resulting score determines which one to engage, and in what order of priority.
In Sales and Marketing, the scores criteria is based on achieving the optimum outcome for the organization. The outcome may be the willingness to buy, customer engagement, profit margin optimization, better loyalty focusing, etc. What lead scoring actually does is to rank qualified prospects or leads against a threshold or barrier. Those leads with scores above this threshold are “qualified” and forwarded from marketing to sales in order to convert the lead to customer.
Regardless of whether it is traditional or predictive, lead scoring is an essential marketing tool, especially when the market is massive and you have limited sales resources to handle such a number of leads.
Traditional lead scoring
Traditional lead scoring makes use of predefined criteria or attributes, multiplied by weighting factors criterion in order to deduce the total score. Criteria definition and weighting factor values depend on the target outcome, with the right choice being the critical points needed to become successful.
Traditional lead scoring attributes
When scoring a lead, the criteria may be distinguished by two categories: explicit or implicit.
Explicit criteria
Often called demographics, explicit criteria are based on characteristics of the lead, and they are intentionally provided by the lead. Explicit criteria may include:
- Industry that the lead belongs to.
- Location.
- Financial size.
- Employee size.
- Contact person job title.
Since such characteristics are not volatile, they are more accurate and reliable.
Implicit attributes
Your implicit criteria are based on behavioral attributes, such as the actions that the lead may take when visiting a B2B website. Contrary to explicit attributes, this information is not deliberately provided by the lead, and it is either extracted or inferred. Implicit attributes may include:
- Online activity such as website pages visited, links clicked, etc.
- Content interaction such as content (brochures, ebooks, etc.) downloads.
- Subscriptions in newsletters, RSS feeds.
A combination of both attribute categories may give the overall score.
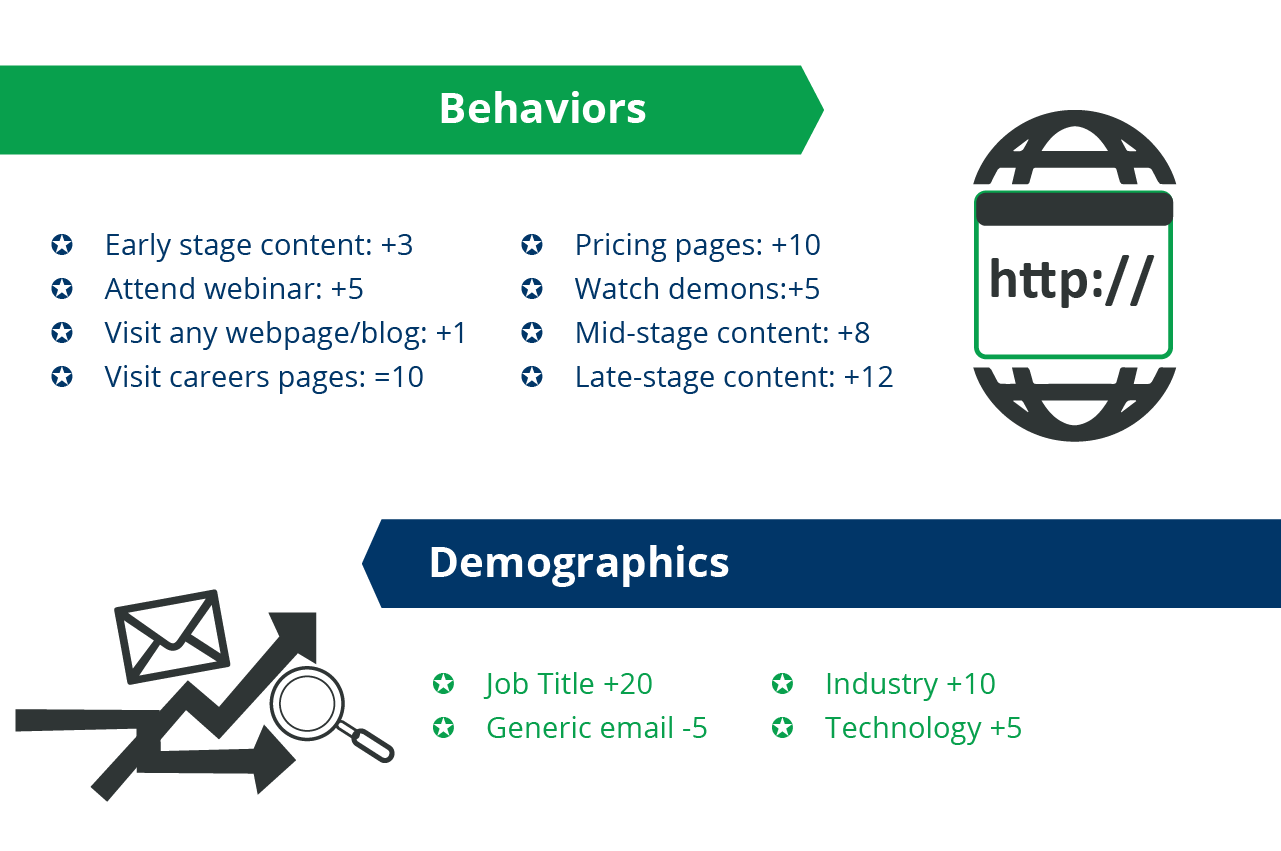
Features and challenges
Consider the following simplified lead scoring system.
At first, you should define the attributes. This may be something custom, based on some common logic (such as negative score for Gmail based email accounts or high score to big size enterprises), or may follow more standardized tools (such as BANT). Once completed, you set the corresponding weighting coefficients and threshold or 'qualified' barrier You then test the model against historical data, distributing it to your sales people if successful.
After each completed sales cycle, you re-evaluate the model and redistribute it back to your sales team until you achieve satisfactory results. You can evaluate the model against successful or failed scorings using procedures based on the simplified rule: A failure is a qualified lead that did not end up as a customer or a non-qualified lead that finally ended up customer.
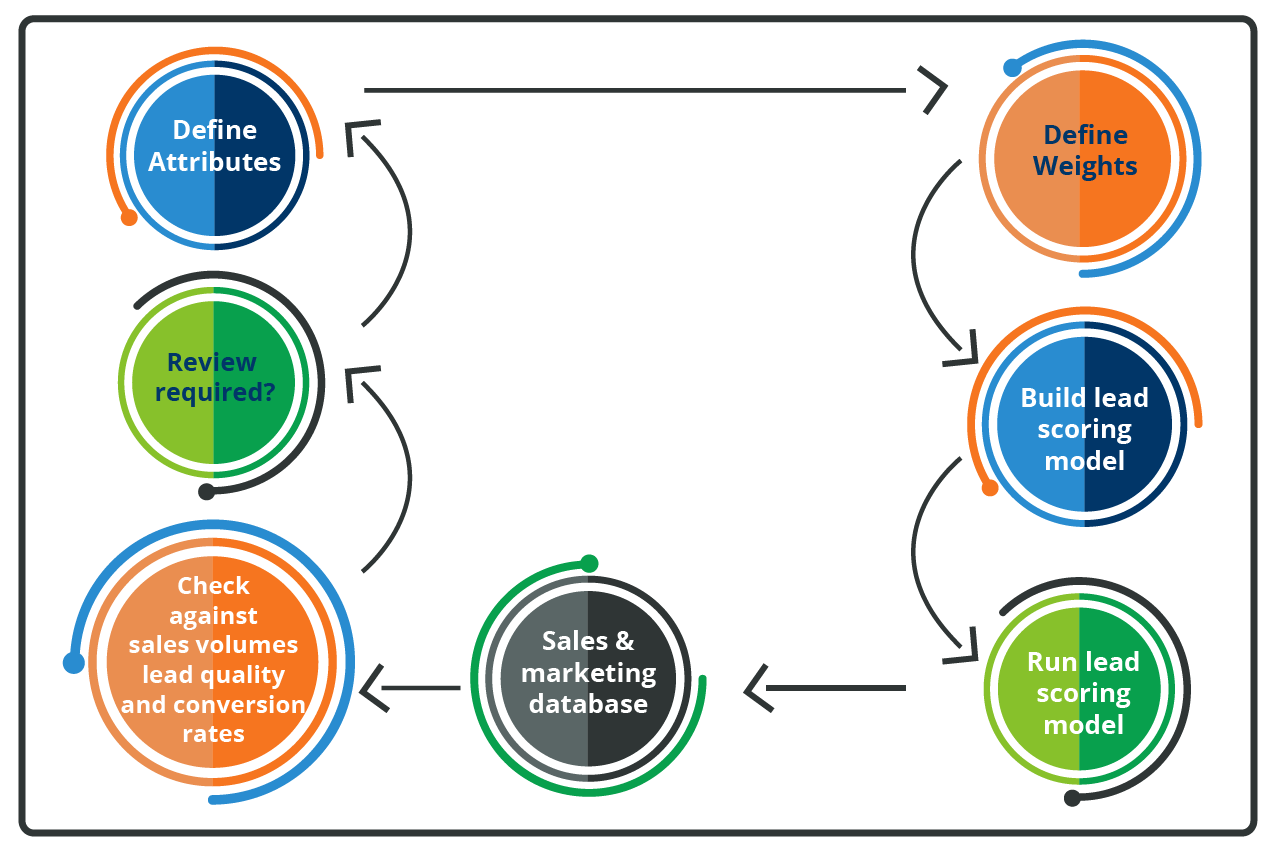
As it easily derived, traditional lead scoring systems are more or less manual systems, in terms of rules definition (attributes and weight factors) and methodology reevaluation improvement and adjustment.
Predictive lead scoring
Despite the fact that traditional lead scoring has been successful for most organizations, 47% of marketers indicate that the quality of leads need improvement and with 43% of them saying that it does not provide sufficient insight into buying attributes. Because of this, predictive lead scoring, has continuously gained acceptance over the last few years:
- Organizations with predictive lead scoring increased 14 times in period 2011-2014.
- 98% of organizations using predictive lead scoring say they would purchase lead scoring again.
Other studies indicate that predictive lead scoring is one of the key factors for increasing qualified leads.
Instead of using rigid rules and scoring a lead on them, predictive lead scoring uses existing sales data, along with data mining and analytics techniques to build the 'ideal' lead. Subsequent leads are compared with this lead and correspondingly labeled qualified or not.
A major innovation in predictive lead scoring is that the marketer does not have to define attributes and their corresponding weight factors. Additionally, there is no need for a periodical 'run and check' process. In predictive lead scoring, the marketer defines the KPIs used in the analytics so the model algorithm can create a formula for automatically ranking leads so the marketer can easily identify the most qualified ones. The model is continuously fed with data on leads that successfully converted to customers or those that failed, eliminating the need for 'run and check' processes.
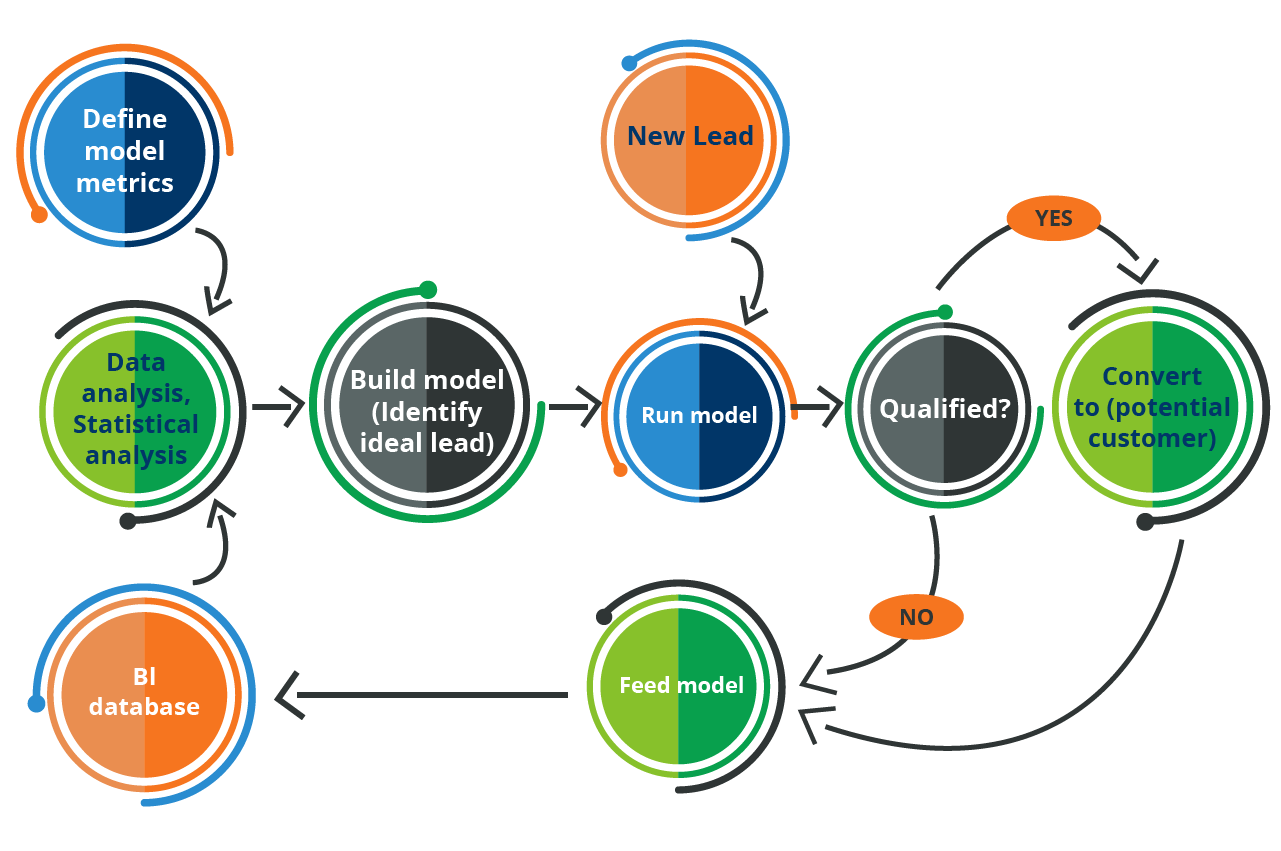
The following table summarizes predictive lead scoring features compared to traditional one:
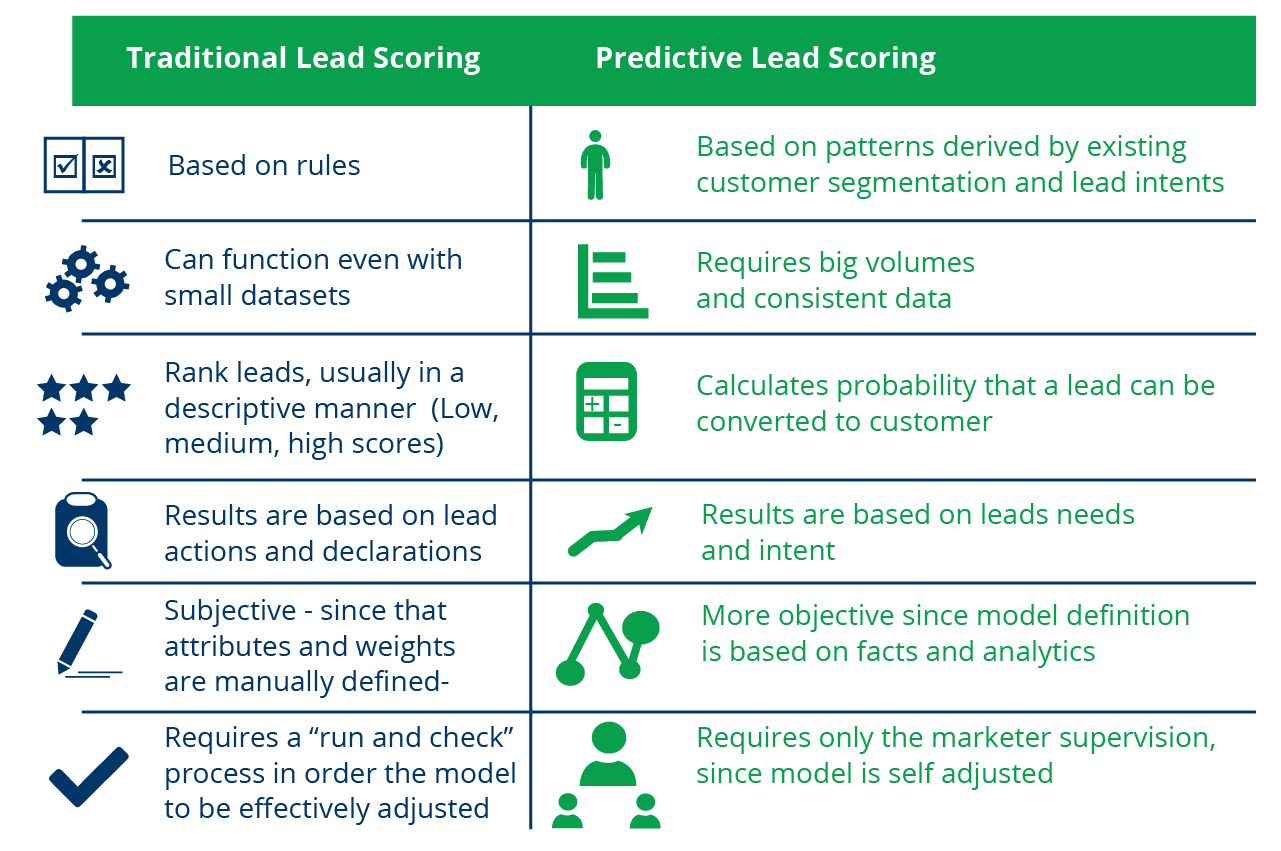
The role of Machine learning and Predictive analysis
Predictive lead scoring uses big data techniques, along with analytics, to build a model to predict the probability a lead will convert to a customer. Perspective analytics may use these probabilities to suggest actions as well as the deliverables and implications. Leveraging predictive and perspective analytics gives the marketers a solid, robust scientific approach on how to effectively increase conversion rates.
Artificial intelligence, in the form of machine learning, is used in predictive lead scoring in several ways:
- In predictive lead scoring analytics, data is not limited to behavior or demographic. You can consolidate and use your own proprietary customer data with public data from providers, social media and other sources, both structured and unstructured as well. Such massive data quantities cannot be analyzed and processed by human intelligence in an acceptable time frame.
- Predictive analysis model feed and review require complicated mathematical and arithmetic computations applied in stochastic and multifactorial systems to operate effectively.
Challenges
Some may expect that moving from traditional lead scoring, or from scratch, to predictive lead scoring requires investments that mid and small size organizations may not be able to afford. Because of this, 83% of organizations using predictive lead scoring are 250M and below, as studies confirm. However, cloud based solutions and vendor partnerships can help organizations to overcome this obstacle.
Another major challenge to predictive lead scoring adoption, compared to traditional, has to do with data:
Not enough data
Predictive analytics require large volumes of data not only to run the model but also to build and test it. While predictive scoring models are designed to be auto fed with new data in both conversion and no-conversion cases, some may not have enough sufficient data available to run the model, especially in the early stages. This is often called a cold start and you cannot reliably make predictions in this situation.
Not consistent data or missing data
You might have large volumes of data but it could be inconsistent and be missing critical information especially when marketing and sales teams are not aligned.
Typical examples of this situation include
- Upon a successful lead conversion, sales representatives do not provide necessary (relative to predictive lead scoring model) information to your organization’s CRM and/or ERP systems.
- Failures to convert information is not posted either inadvertently or on-purpose.
Setting up the right qualification criteria for the model
Provided that the data is available and of good quality, the model can set up basic qualification criteria by itself. The marketer should not consider predictive lead scoring as a “black box”, but should continuously evaluate and adjust its criteria.
Sales teams are not on board
Predictive lead scoring helps align the marketing and sales teams since it produces qualified leads of good quality and large volumes. Thus, you need to educate your sales team on how predictive lead scoring works, what goes into it, and why it’s been scored that way. If this is not the case, they will continue to use the old way of conversion, ignoring the predictive model. Credibility of the model and education of sales is key.
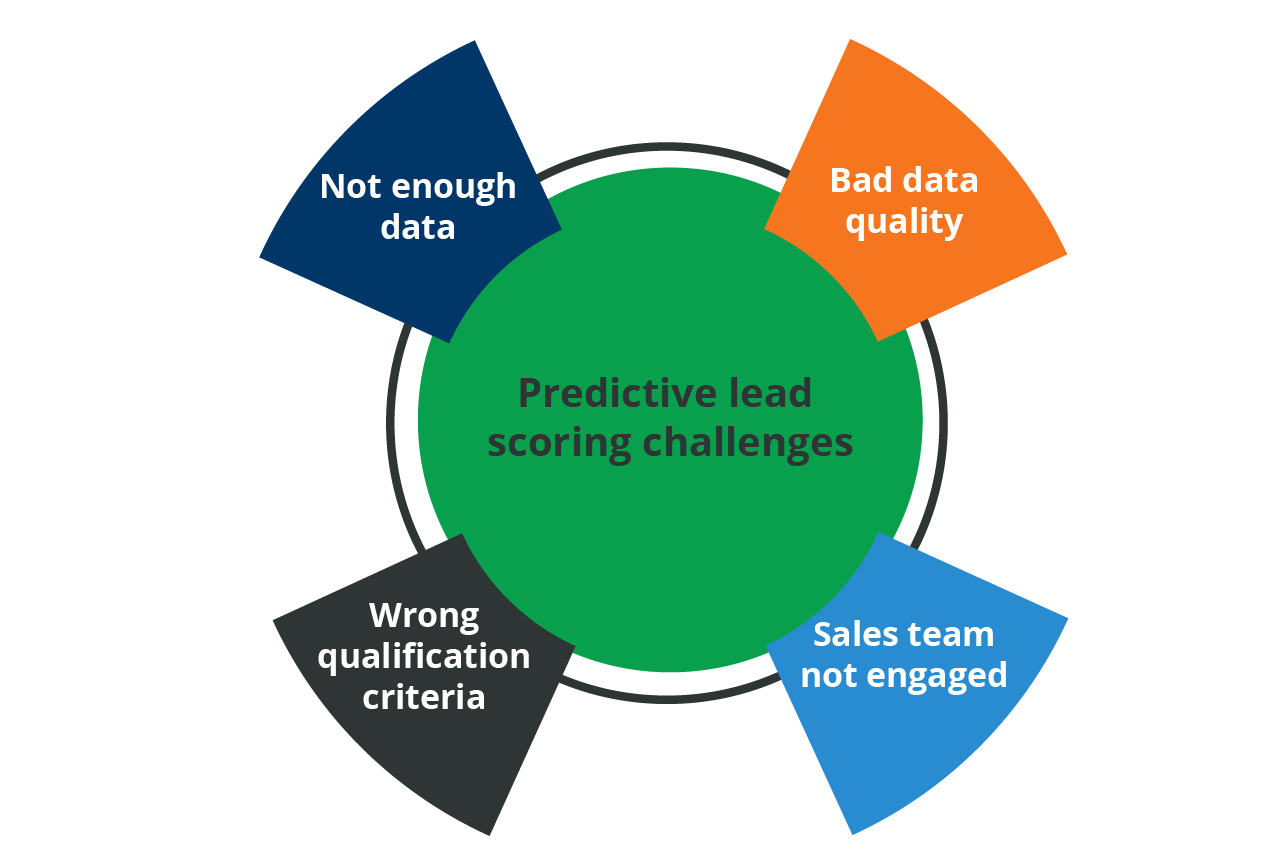
Conclusion
Although a relatively new methodology, predictive lead scoring has been proven effective and reliable for turning properly qualified leads into customers. Based on artificial intelligence capabilities, it has been one of the key factors for marketing automation.
Leave a Comment